Sparking innovation | High-Entropy Alloys in electrocatalyst optimization
In our previous single-atom catalyst blogpost, we explored well-defined catalysts in existence: the single-atom catalyst showing highest atom efficiency that can efficiently participate in the reaction.
In this blog, our focus shifts towards exploring the use of high-entropy alloys (HEAs) in electrocatalyst optimization. We will also explore ways that result in the identification of a good catalyst through closed loop optimization: a process that requires no human intervention and automatically adjusts material properties through machine learning (ML) models.
In the realm of electrocatalyst optimization, i.e. finding the right catalyst to do the job, the traditional approach has long relied on iterative empirical methods: endless cycles of testing, learning, and repeating—a process notorious for its sluggish pace. Yet there is a significant urgency to propel electrocatalysts, the catalysts that facilitate the conversion of reactants such as CO2, N2, and H2O into valuable products such as hydrogen, carbon fuels, and chemicals powered by electricity. Therefore, a definitive energy transition demands a paradigm shift towards accelerating this optimization process. [1]
Accelerating electrocatalyst optimization through machine-learning informed synthesis
One promising avenue poised to revolutionize the landscape of electrocatalyst screening lies in the integration of machine-learning-based informed synthesis feedback loops. Through the synthesis, characterization, and testing of electrocatalyst libraries, structure-activity relationships can be learned by a machine-learning model. Subsequently, the model provides a roadmap for the next batch of experiments aimed at optimizing the reaction. With each iteration, the machine learning (ML) model enhances its predictability, thereby facilitating significantly accelerated optimization (Figure 1). As commonly acknowledged, machine learning excels at interpolation rather than extrapolation. Therefore, it is crucial to select a candidate catalyst library that plays to the strengths of ML-based optimization schemes, not its weaknesses.
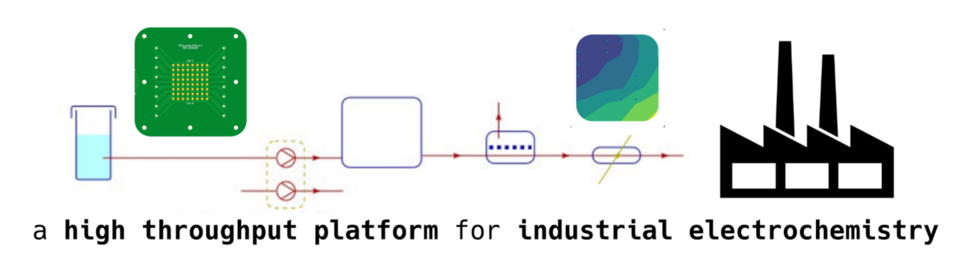
Harnessing the power of High-Entropy Alloys: flexibility, stability, and performance
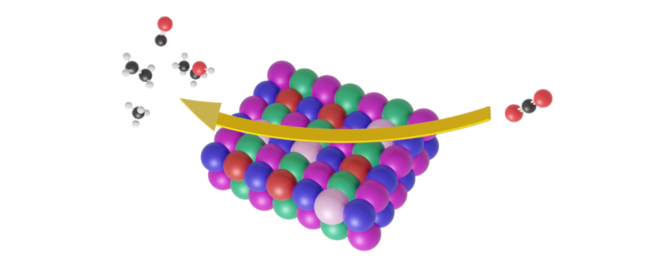
The triumph of entropy: A homogeneous blend
Nanoparticles have emerged as essential components in material design, and further alloying multiple elements into nanoparticles proves to be a productive approach for both research and industrial purposes. [2] Enter the realm of high-entropy alloys (HEAs), a captivating candidate system consisting of nanoparticles boasting five elements or more. [3] [4] HEA nanoparticles demonstrate an exceptional quality: they ensure a homogenous mixture of elements, regardless of the individual miscibility of their components in macroscopic systems (their tendency to mix or unmix, similar to the concept of mixing oil-in-water vs alcohol-in-water). Their stability is owed to the triumph of entropy (a measure of disorder) over the enthalpy term (a measure of order). This is a profound feature of HEAs as their synthesis guarantees a catalyst library to which the interpolation principle holds: each composition produced is a combination of its individual components arranged in a random fashion. That way, a series of catalysts can be made suitable for ML-informed performance optimization. Hence, HEAs can offer a surface with an exceptionally vast array of unique binding site environments, resulting in a nearly continuous distribution in the configurational space (Figure 2).
Unlocking a vast configurational space
HEA nanoparticles grant access to a vast configurational space: different choices of arrangements of the different elements. All these different arrangements, or configurations, result in slightly different catalyst active sites that have the potential to activate the inert molecules such as H2O, CO2, N2, and O2 we will need to convert to power in the future. Further, it enhances the stability of the material's phase as there is a strong resistance against unmixing. This extends the catalyst lifespan and can even reduce corrosion.
Navigating a formidable challenge
However, amidst the promise HEAs harbor, lies a formidable challenge—the vastness of the configurational space. Consider a HEA nanoparticle alloy comprising 5 components and housing hundreds of surface atoms. It harbors over 10^20 unique configurations! To navigate this extensive compositional expanse, researchers have turned to the realm of machine learning, harnessing its capabilities to train models that discern the most promising structures from this vast array. [5] [6] However, despite the acceleration and precision afforded by these informed decision-making processes, the rapid production of HEAs to validate or challenge predictions and refine accuracy poses a formidable challenge, emerging as a critical bottleneck. Conventional methods of producing HEAs from their bulk counterparts, such as ball milling of individual components or employing wet-synthesis and carbothermal shock synthesis techniques, yield a single composition per batch. Such constraints starkly contrast with the high-throughput demands necessitated by the machine learning-driven exploration of high-entropy alloy compositional space. How does one devise a synthesis system sufficiently agile to quickly alter, test, and integrate the compositions of produced HEAs and their performance into the ML algorithm, thereby accelerating the test-learn-repeat cycle?
VSParticle's Nanoprinter: Revolutionizing synthesis for multi-component materials
VSParticle is a pioneer in the field of (nano)particle generation and printing. The company’s flagship product, VSP-P1, enables fast and easy synthesis and printing of (semi-) conductive nanoporous layers for various applications (Figure 3).
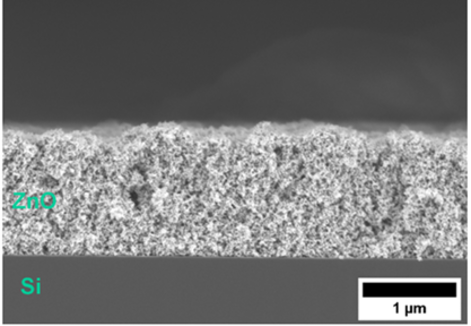
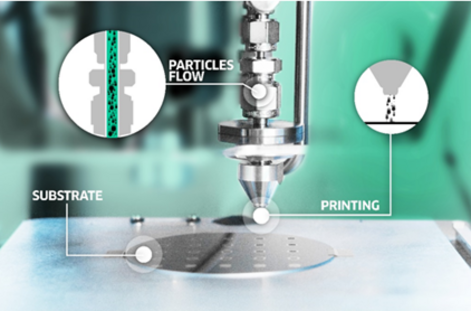
The VSP-P1 Nanoprinter, coupled with VSP-G1 nanoparticle generators, is poised to overcome this challenge with ease. This innovative technology uses spark ablation to generate particle beams of one or more pure elements that seamlessly mix in the aerosol stream, facilitating the production of nanoparticles containing quintuple compositions (Figure 4). Furthermore, the ability to finely tune the relative power of each spark generator offers unparalleled control, enabling instantaneous alterations in nanoparticle compositions—a feat as effortless as pushing a button.
In addition to nanoparticle generation, VSP-P1 Nanoprinter effortlessly transforms HEA nanoparticles into intricate nanoporous thin films. Through its user interface, users can deposit HEA nanoparticles into a matrix of nanoporous layers with varying compositions and properties on substrates. This automation also enables users to customize printing conditions to be tailored from spot to spot, eliminating the need for manual intervention at every step.
From nanoparticles to High-Entropy Alloys: Exploring the path to enhanced catalyst performance
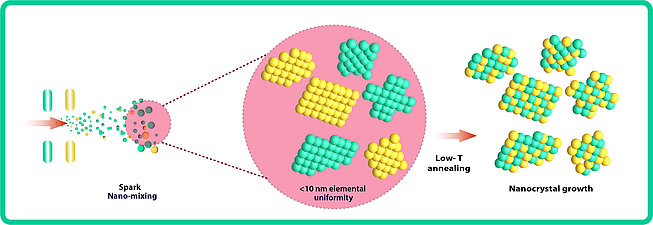
One question remains unanswered, however: can nanoparticles comprising five components, synthesized via spark ablation, be seamlessly transformed into HEAs? Recent demonstrations by VSParticle customers offer the following: the perturbation of bimetallic nanoparticles with a thermal treatment can induce alloying if they are small enough (Figure 5). [7] Moreover, assisted by the favorability of entropy in HEAs, this transformation promises to be even more facile. The HEA vast material space made accessible with the VSP-P1 offers an exemplary route to fast catalyst screening and closed-loop optimization schemes.
VSParticle aims to occupy the space of high-throughput experimentation in which automated closed-loop catalyst optimization schemes are used to vastly accelerate catalyst discovery and optimization.
By leveraging HEAs' unique configurational space and ML's predictive power, we can rapidly iterate towards catalysts with enhanced electrochemical performance. High-throughput research gains significantly more value when the tested materials can be manufactured in larger quantities. Therefore, VSParticle's Nanoprinter offers facile synthesis capabilities catering to the extensive compositional range of HEAs, delivering rapid and reproducible results. This technology uses thermal treatment to process a vast number of element combinations by applying multiple sparks per element for industrial production and one spark per element for discovery. With this innovative approach, VSParticle is developing new generations of printer technology to scale catalyst production to industrially relevant capacities and facilitate sustainable energy solutions that are vital for a greener future.
For more information about VSParticle’s technology and to explore the possibilities in your field, please get in touch with us.
Acknowledgment
Special thanks to Cedric David Koolen, Principal Investigator @EPFL, for sharing his knowledge and expertise.
References
[1] R. W. K. P. T. V. K. J. v. &. S. K. J. Becker, "A Scalable High-Throughput Deposition and Screening Setup Relevant to Industrial Electrocatalysis," Catalysts, vol. 10, p. 1165, 2020.
[2] D. C. P. P. Y. J. J. Y. M. C. J. Feng, "Unconventional Alloys Confined in Nanoparticles: Building Blocks for New Matter," Matter, vol. 3, pp. 1646-1663, 2020.
[3] T. e. a. Löffler, "Toward a Paradigm Shift in Electrocatalysis Using Complex Solid Solution Nanoparticles," ACS Energy Lett, vol. 4, p. 1206–1214, 2019.
[4] T. A. A. e. a. Batchelor, "High-Entropy Alloys as a Discovery Platform for Electrocatalysis," Joule, vol. 3, p. 834–845, 2019.
[5] T. A. A. B. A. B. a. J. R. Jack K. Pedersen, " High-Entropy Alloys as Catalysts for the CO2 and CO Reduction Reactions," ACS Catalysis, vol. 10(3), p. 2169–2176, 2020.
[6] C. M. R. J. &. U. Z. W. Clausen, "Adapting OC20-trained EquiformerV2 Models for High-Entropy Materials," Materials Science, 2024.
[7] H. e. a. Dai, "Sub-10 nm Mixing and Alloying of Cu–Ag and Cu–Ni via Accelerated Solid Diffusion," ACS Appl. Mater. Interfaces, 2023.
Comments
No Comments