High throughput experimentation for material discovery
In this blog, we will talk about accelerating material development using state-of-the-art technology that is the basis of high throughput platform to synthesize novel materials to support the ongoing effort of optimizing electrolysers. Currently, to test these materials, classical single-fold test technology is mainly used for electrochemical R&D in electrolysis or fuel cell applications. However, electrocatalysis requires more advanced digitalization tools as the parameter space expands by numerous new electric parameters. It seems likely that the current tools will no longer be enough and must be upgraded by applying feedback loops, machine learning, or a combination of the two. We use a case study to illustrate the key features of high throughput experimentation and screening in the electrocatalyst space.
Urgency in accelerating material development
In order to reach a 55% reduction in net GHG emissions by 2030 and achieve climate neutrality by 2050[1], hydrogen technologies will play a vital role in Europe in the coming decades. In particular, water electrolysis will become a key technology for producing green hydrogen.
To effectively tackle the climate crisis, we must rapidly transition to renewable energy sources like solar and wind. These sources are intermittent, meaning they only produce electricity when the sun shines and the wind blows. This variable electricity generation does not guarantee the security of the energy supply. To ensure a stable energy supply, we need efficient methods to store surplus energy and release it when energy demand is high or when these intermittent energy sources are unavailable. Hydrogen provides that needed flexibility in the energy system. It can be produced through water electrolysis using renewable electricity in a clean and environmentally sustainable manner during periods of excess energy production. It can be easily stored and later used in fuel cells to generate electricity.
Despite its potential, this approach has yet to be widely adopted due to high costs. The processes involved in hydrogen production and utilization rely on expensive noble metal-based catalysts, an essential component of the electrolyser stack. Therefore, innovations in sustainable material development are crucial for the future of renewable energy, energy storage, and sustainability to realize the full potential of hydrogen as a sustainable energy solution. This requires strong R&D support with enhanced experimentation and testing technology.
Why does it take 15 years to market novel materials?
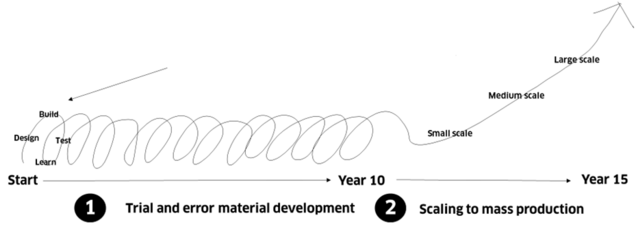
Traditional methods of discovering new material needs acceleration in an efficient manner to be more sustainable. If not, synthesis and testing for novel materials would require immense resources (i.e. human labour and machinery) and time until an optimal material would be found. This trial-and-error process requires hundreds or thousands of iterations to identify the best catalyst, resulting in a timeline extending up to 15 years from initial discovery to mass production [2] (figure 1). Given the urgent need to address climate change, such delays are untenable. Therefore, it is crucial to redesign every step in developing new materials to get the shortest time from lab-to-fab.
Evolving landscape of high throughput experimentation
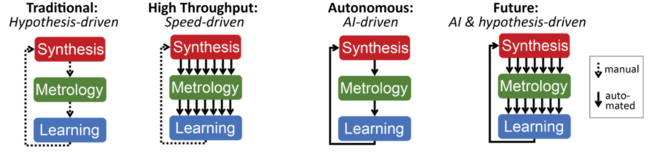
As a first step in speeding up, scientists are adopting alternative techniques for material discovery, such as high throughput experimentation (HTE). In this method, a looping system is continuously iterated through establishing a gradient library of materials by a computational model, then their synthesis and finally testing. The value of HTE at this point is to couple with a machine learning (ML) algorithm so that the simultaneous execution of numerous experiments would be optimized for the best material and enhanced for the highest reproducibility through automation. So, HTE surpasses traditional methods by significantly increasing speed and efficiency to generate a comprehensive data sets at minimum cost and resource use.
Herein, the combination of machine learning (ML)- based algorithms with HTE has surfaced as a powerful tool to speed up the exploration of chemical space. HTE has been used to quickly generate high-quality datasets to feed ML algorithms cost-effectively and to quickly find suitable initial reactivity points to further optimize them using ML approaches.
Unlike traditional methods that rely on parallelization, these systems leverage AI-guided decision-making to optimize the selection of experiments. This shift aims to accelerate knowledge acquisition by prioritizing the most promising experiments, thus allowing self-driving labs (SDLs) to efficiently explore vast experimental spaces more efficiently towards material discovery.
VSParticle collaboration to advance HTE
Earlier this year, VSParticle did a collaborative project with Caltech (California Institute of Technology) showcasing promising results supporting advancement in HTE. The findings suggest that existing high throughput electrochemical screening techniques can be successfully applied to explore printed nanoparticle arrays rapidly. This validation marks a significant step forward in the ability to control synthesis parameters and optimize nanoparticle compositions for various applications through automated VSP-P1 nanoprinter.
Using the VSParticle nanotechnology, Pt-Ni nanoporous thin films of varying compositions were deposited on an Indium Tin Oxide coated (ITO) glass plate, demonstrating independent control of elemental composition and patterning, loading, and nanostructuring. Caltech's high-throughput screening of these VSP's Pt-Ni sample arrays for the Oxygen Reduction reaction (ORR) activity showcased superior comparable performance compared to traditional combinatorial libraries synthesised by conventional other methods with longer and tedious synthesis protocols. A significant proof of concept activity was demonstrated to lower the precious metal loading in fuel-cells and electrolysers owing to 50:50 Pt:Ni gave a similar activity to pure Pt. Meanwhile, the low onset potential for ORR (1 V vs. RHE) hints fast kinetics thanks to the highly active surface area of VSP's nanoparticle film structure. To ensure good reproducibility, these samples were also validated for reproducibility by testing duplicates of each composition.
These results hint that VSParticle nanoprinting technology is a suitable candidate aims to accelerate every step of the material development process from nanograms to kilograms, via fundamental discovery to high throughput screening and then to mass production. This acceleration of synthesis-deposition-testing cycle is paramount to investigate thousands of catalysts in a single day. By doing so, i.e. impacting every step of the process, only then the overall time of 15 years could be reduced to a year.
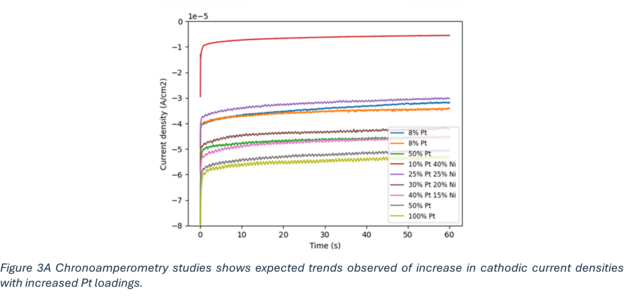
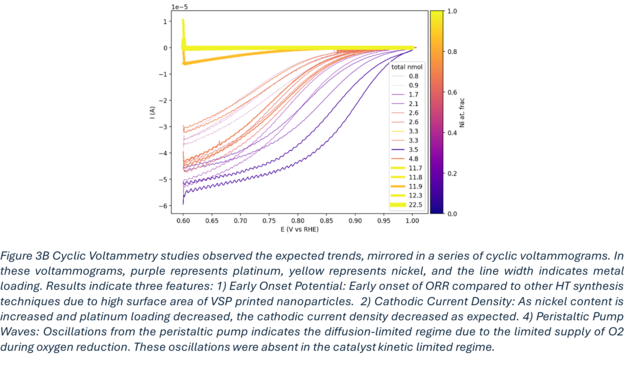
The ‘trough of disillusionment’ & role of Artificial Intelligence (AI)
Despite these advancements, the field encountered a "trough of disillusionment" after the initial surge in excitement. One contributing factor is the sheer number of potential experiments. For example, addressing a problem involving a three-element system could theoretically require billions of experiments. Even with a thousand-fold increase in throughput, the research cycle still spans years, leaving vast experimental spaces largely unexplored. To overcome these challenges, integrating artificial intelligence (AI) to guide our experimental design is critical. AI can needs to be designed for helping researchers to navigate the immense experimental space by making informed decisions about which experiments to conduct next for the most efficient process route.
The future of HTE
As stated earlier, considerable progress has been made in automating experimental workflows in materials science. However, a vast "ocean of opportunity" remains to enhance AI's reasoning and planning capabilities.
A crucial distinction between automated systems and human scientists is the ability to exercise intuition and adaptive reasoning. Human researchers don't merely follow a pre-defined experimental plan; they continuously adapt based on observations and prior knowledge. They can identify surprising results and pivot their research direction accordingly. Emulating this level of dynamic decision-making in AI remains a significant challenge.
In conclusion, HTE has come a long way, from manual processes to automation and now to AI-guided systems. While challenges remain, particularly in emulating human intuition, the future holds immense potential for further advancements. By continuing to integrate AI and automation, and fostering strong collaborations, we can unlock new frontiers in materials discovery and accelerate scientific progress.
Stay tuned for our next blog where we will discuss the significance of Self-Driving Labs (SDLs) and ground-breaking initiatives that are advancing their evolution.
Acknowledgment
Special thanks to Nayab Azam (Technical Content Writer), Erdem Irtem (Application Specialist, Electrochemistry), and Anagha Vijayakumar (Product manager SDL) for contributing and sharing their knowledge and expertise in writing this blog post.
References
[1] 2050 long-term strategy - European Commission (europa.eu)
[2] Gregoire, J.M., Zhou, L. & Haber, J.A. “Combinatorial synthesis for AI-driven materials discovery.” Nature Synthesis (2023): 493–504.
Comments
No Comments