Self-Driving Labs Transforming Material Research
In our previous blog we talked about urgency of material discovery in energy sector and importance of high throughput experimentation in self-driving labs (SDLs). In this blog, we discuss the significance of SDLs and ground-breaking initiatives that are advancing their evolution. We also explain how VSParticle technology offers a robust foundation for future SDLs. The blog also explores the relevance of decentralisation and open sourcing and how these concepts are revolutionizing the pace of material discovery.
SDLs: A game-changer in scientific research
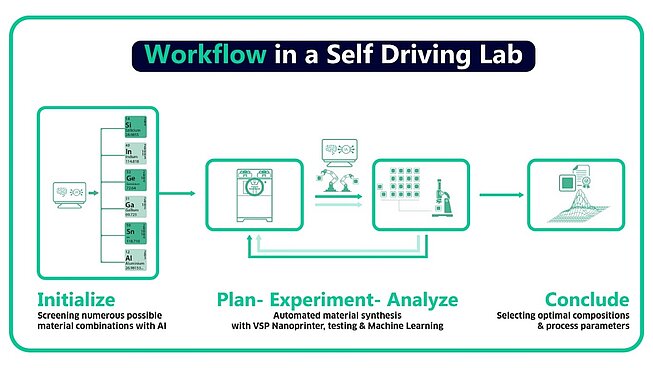
Self-driving labs (SDLs) revolutionize the combinatorial approach by combining robotics, artificial intelligence, and machine learning to automate and optimize experiments. A SDL is simply combining the best technologies of this time. This integration of best technologies promises to substantially accelerate the rate of materials discovery by 100 times, surpassing human capabilities alone.
In SDLs, data is collected through high-throughput experimentation which is fed into an AI system. The AI system learns from the results and predicts improved material candidates with desired properties for specific applications. Robotic platforms then autonomously test these predictions by synthesizing and evaluating the materials. The integration of data, computation and experiments is essential with each element guiding and refining the others in a feedback loop (figure 1).
In this data-rich paradigm, the ability to rapidly produce and analyze large volumes of experimental data is foundational for driving meaningful progress and innovation. The more experiments performed, the faster the AI is able to learn. So, an ideal SDL inverts the traditional discovery process, allowing scientists to define desired properties first and then work backwards to develop new materials without the tedious hours of trial and error typically required in the lab. VSParticle’s nano-printers are an integral part of SDLs enabling automated material synthesis thus supporting high through put experimentation.
The VSP-P1 nanoprinter has already been adopted by pioneering research groups at top institutes, including UL Research Institute and DIFFER. By enabling the integration of our synthesis module into SDLs, our technology empowers these researchers and industries to accelerate material discovery processes in various fields such as gas sensing, catalysis, electrocatalysis.
Initiatives pioneering SDLs
There are numerous noteworthy initiatives across the globe that are pioneering SDLs. Despite being in the early stages of development, several SDLs are already showing impressive results that not only accelerate the discovery of materials but also streamline the research process, significantly advancing the development of innovative energy materials. We provide an overview of some of these multi-lateral collaborations involving research institutes, universities and industry leaders that are investing efforts to advance innovation in this space.
Initiatives towards SDLs | ||
---|---|---|
Research centres and institutes | Leadership | Scope of work |
Helmholtz Institute Erlangen – Nuremberg (HIERN) | Dr. Dominik Dworschak [1] Dr. Serhiy Cherevko [2] | Utilizing high-throughput synthesis methods such as automated solution mixing, drop casting, physical vapor deposition, and electrodeposition to rapidly create diverse catalyst libraries. These materials undergo automated physicochemical characterization and swift electrochemical screening using advanced electrochemical scanning flow cell (SFC) techniques. The integration of large datasets with data science tools, including machine learning and Bayesian Optimization, facilitates efficient data interpretation and iterative experimental planning. |
Materials Discovery Research Institute (MDRI) [3] – UL Research Institutes | Dr. Stuart Miller [4] Vice president and Executive Director | Focusses on developing new, cost-effective, and safer alternative fuels and storage solutions. The team at MDRI leverages the synergy between advanced computing and high-throughput experimental methods to drive innovation. |
Catalytic and Electrochemical Processes for Energy Applications (CEPEA)[5] – DIFFER | Dr. Mihalis Tsampas Group leader | Develops and utilizes automated virtual materials discovery frameworks, leveraging high-throughput physics-based classical and quantum calculations, artificial intelligence methods, and advanced data infrastructures. |
Autonomous Energy Materials Discovery (AMD)[6] – DIFFER | Dr. Süleyman Er Department Head and Group leader | Focuses on electrochemical membrane reactors for producing solar fuels and electrifying the chemical industry. In materials synthesis, the team employs nanostructuring techniques to increase the active surface area of electrocatalysts and enable atomic-level control of exposed sites. |
Autonomous Discovery of Alloys (AUTODIAL) – University of Toronto | Jason Hattrick-Simpers [7] Professor, University of Toronto | The team utilises automated experimental platforms and AI to explore high entropy alloys, metallic glasses, intermetallics, and ceramic coatings, collaborating with industrial and national labs to accelerate transition from lab to industry. They develop autonomous materials science tools to accelerate the discovery of new materials, integrating AI and automation to streamline synthesis and characterization. Their work also enhances scientific AI by combining traditional modeling with AI, optimizing and discovering materials while deepening the understanding of their behavior. Committed to democratizing science, they share all data, code, and protocols publicly to empower global scientific inquiry. |
Project Ada – University of British Columbia [8] | Alán Aspuru-Guzik [9] Curtis Berlinguette [10] Jason Hein [11] | This lab fast-tracks materials for solar cells, fuel cells, and thermoelectric. Ada stands out as the first fully automated lab using inorganic powders as starting ingredients. Through this solid-state synthesis approach a wide variety of materials can be used producing large quantities suitable for application. |
SmartDope – North Carolina State University (NCSU) [12] | Milad Abolhasani [13]
| An autonomous lab focused on developing quantum dots with the highest "quantum yield," which measures efficiency in converting absorbed photons into emitted ones. |
Jensen Research Group - Massachusetts Institute of Technology (MIT) [14] | Klavs F. Jensen [15] Timothy F. Jamison [16] Connor W. Coley [17] | Developed an advanced automated laboratory system that significantly streamlines the production of small molecules, potentially revolutionizing fields such as medicine, solar energy, and polymer chemistry. This innovative system integrates AI-guided software, expert chemist oversight, and a robotic platform to automate the entire molecular synthesis process. |
Consortiums | ||
Research groups and institutes | Leadership | Scope of work |
Acceleration Consortium [18] and CAPeX Pioneer Centre for Accelerating P2X Materials Discovery [19] | Tejs Vegge [20] Alán Aspuru-Guzik [21] Director Acceleration Consortium | University of Toronto secured a $200-million grant from the Canada First Research Excellence Fund (CFREF) to transform the pace and effectiveness of scientific discovery through its Acceleration Consortium. This grant will aid the consortium's development of "self-driving labs," integrating advanced technologies to expedite the discovery of new materials and molecules, significantly reducing the usual time and cost involved. Together with CAPeX, these consortiums emphasize collaboration among academic, governmental, and industrial partners to accelerate innovation, and optimize resource utilization. Their focus includes developing scalable and efficient materials for clean energy to drive transformative scientific breakthroughs. |
Open Catalyst project[22] | Fundamental AI Research (FAIR) at Meta AI Carnegie Mellon University's (CMU) Department of Chemical Engineering | The aim is to discover affordable catalysts for converting excess solar and wind energy into alternative fuels. Open Catalyst aims to screen millions, even billions of catalysts annually using a combination of quantum mechanical simulations and machine learning. |
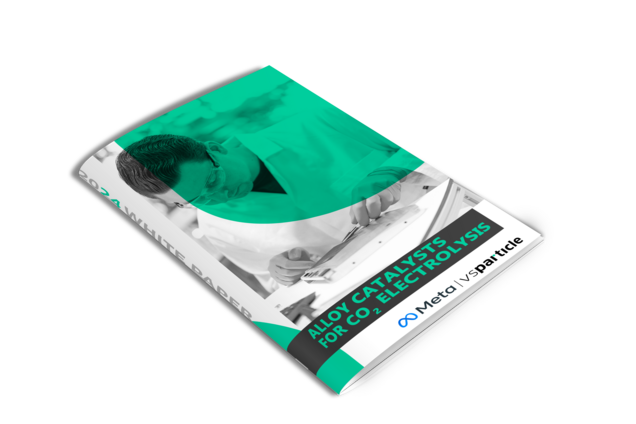
Get access to detailed analysis of alloy catalysts datasets synthesized with VSParticle technology for Meta's Open Catalyst Experiments 2024
Decentralization and open-sourcing data
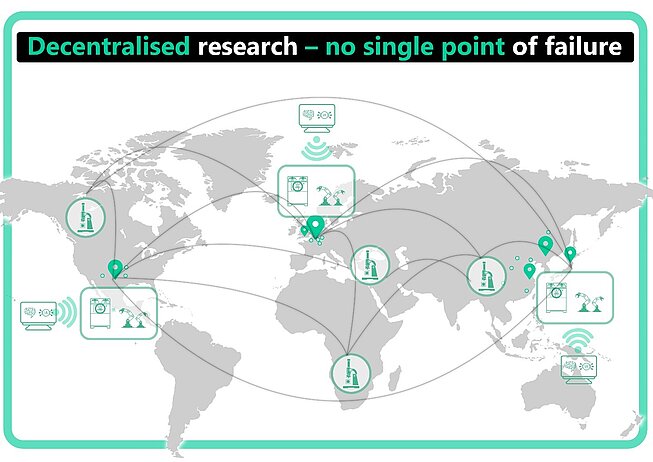
Traditional research methodologies are often centralized and lack comprehensive data sharing leading to redundant experiments. Research findings obtained at a small lab scale frequently fail to translate into practical applications, hindering progress. Consequently, billions of dollars are wasted on duplicative research efforts worldwide. Decentralizing research activities and open-sourcing data can address these challenges. In a decentralized network, there is no single point of failure (as shown in Figure 2). If one part encounters issues, others can continue functioning, maintaining overall research momentum. This redundancy ensures that scientific progress is not halted by localized problems. Open source allows material developers to modify, customize and build upon existing candidate designs. This flexibility enables researchers to experiment with new ideas without starting from scratch.
Open-sourcing data is another critical component of this new paradigm. By sharing data freely, researchers can avoid redundant efforts and build upon each other’s work more effectively. High throughput labs generate large sets of data which can be analysed more efficiently through collaborative efforts across organizations. META AI exemplifies how open-sourcing data can revolutionize the pace of discovery. By providing a platform for sharing experimental data, META facilitates collaboration and accelerates innovation.
As Jason Hattrick Simpers, Professor at University of Toronto, puts it "The aim is not to run a 100,000 or billion experiments, but to perform the most informative experiments at any given time using concepts like active learning."
VSParticle – a robust foundation for SDLs
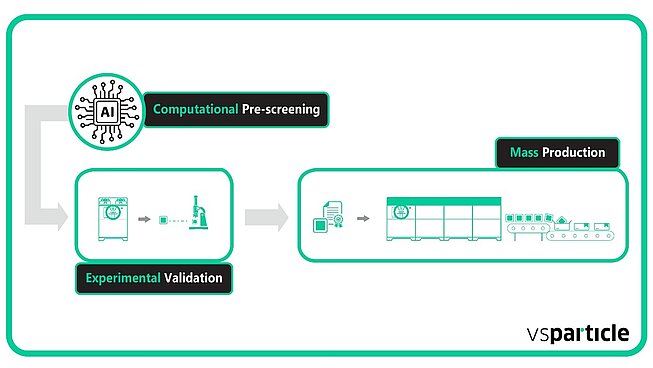
SDLs represent a significant leap forward, combining AI, robotics, and high-throughput experimentation to rapidly discover and optimize new materials. Decentralization and open-sourcing of research data further enhance the potential of SDLs, fostering collaboration and ensuring that advancements can be built upon globally.
While AI significantly speeds up the discovery and validation of new materials, the absence of a seamless method to scale these innovations from the lab to industrial production, hinders their practical application and limits the value of AI-based research. VSParticle offers a robust and scalable tool that bridges this critical gap by offering robust and scalable tool that can facilitate this transition from research to industrial production, ensuring that the promising materials identified through AI can be efficiently produced on a large scale.
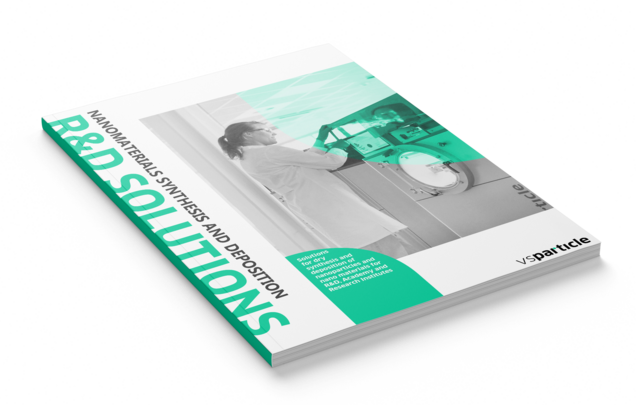
Discover what’s possible with our nano printing technology
Acknowledgment
Special thanks to Anagha Vijayakumar (Product Manager SDL), Aaike van Vugt (CEO) , and Nayab Azam (Technical Content Writer) for contributing and sharing their knowledge and expertise in writing this blog post.
References
[1] Serhiy - Electrocatalysis group
[2] Dominik HT group
[3] MDRI
[4] About Us | UL Research Institutes
[5] CEPEA
[6] AMD-DIFFER
[7] Jason Hattrick-Simpers - Department of Materials Science & Engineering (utoronto.ca)
[8] Home - Ada (projectada.ca)
[9] Alán Aspuru-Guzik | Department of Chemistry (utoronto.ca)
[10] Curtis Berlinguette | UBC Chemistry
[11] Jason Hein | UBC Chemistry
[12] Flow Chemistry and Microfluidics (abolhasanilab.com)
[13] Milad Abolhasani | Department of Chemical and Biomolecular Engineering (ncsu.edu)
[14] MIT - Jensen group
[15] Klavs F. Jensen – MIT Chemical Engineering
[16] Timothy F. Jamison – MIT Department of Chemistry
[17] Connor W. Coley – MIT Chemical Engineering
[19] CAPEX-DTU
[20] Tejs Vegge — Welcome to DTU Research Database
[21] Acceleration Consortium (utoronto.ca)
Hattrick-Simpers, Jason, et al. "Designing durable, sustainable, high-performance materials for clean energy infrastructure." Cell Reports Physical Science 4.1 (2023).
Volk, Amanda A., and Milad Abolhasani. "Performance metrics to unleash the power of self-driving labs in chemistry and materials science." Nature Communications 15.1 (2024): 1378.
Guevarra, Dan, et al. "Orchestrating nimble experiments across interconnected labs." Digital Discovery 2.6 (2023): 1806-1812.
Comments
No Comments